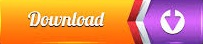
#Imagezilla lsq free
"In addition to that, buyers can greatly reduce costs and free up valuable resources to focus on more strategic initiatives." LSQ FastTrack can provide same-day payments to keep suppliers happy and financially viable. "For most companies, resources are constrained," said Shah, "and that impacts the ability to ensure payment certainty for their suppliers, which puts a strain on the business relationship. "By combining the ease of our accounts payable finance options with the ability to simplify and automate all payments, buyers now have a comprehensive solution to offer early payments to all their suppliers so that they can retain competitive advantage and continue to strengthen their supplier relationships."Īccording to Shah, the new capabilities greatly reduce the burden on accounts payable and procurement teams and can increase the efficacy of a supply chain finance or dynamic discounting program at scale with all suppliers globally. "When we looked at the supply chain finance and payments solutions available, we realized the current offerings didn't address the last mile when solving for working capital," said LSQ Chief Revenue Officer Vikas Shah. For invoices that are not selected for early payment and have reached maturity, LSQ enables routing of funds directly to the supplier from a for-benefit-of (FBO) account.Īll without day-to-day input from the buyer or commitment of technical resources. The platform sends supplier payments from LSQ for early-paid invoices as part of a supply chain finance program. LSQ FastTrack payments management routes funds to suppliers based on when payment is made. With these new FastTrack payment features, businesses on the platform can fully automate their payments processes across all payment methods while giving suppliers payment certainty whether they are paid early or at terms. I will be glad to join the discussion.ORLANDO, Fla., Ap/PRNewswire/ - LSQ, a leading provider of working capital finance and payments solutions, announces the launch of payments management as part of its LSQ FastTrack® platform for corporate buyers.
#Imagezilla lsq code
However, if you find any bugs in my code or have any ideas to improve the quantization results, please feel free to open an issue. Thus, I may not spend too much time continuing to optimize its accuracy.
#Imagezilla lsq professional
I am not a professional algorithm researcher, and I only have very limited GPU resources. The quantization framework will automatically replace layers specified in quan/func.py with their quantized versions automatically. Thanks to the non-invasive nature of the framework, it is easy to add another new architectures beside ResNet.Īll you need is to paste your model code into the model folder, and then add a corresponding entry in the model/model.py. Supported Modelsįor the ImageNet dataset, the ResNet-18/34/50/101/152 models are copied from the torchvision model zoo.įor the CIFAR10 dataset, the models are modified based on Yerlan Idelbayev's contribution, including ResNet-20/32/44/56/110/1202. In my implementation, the step sizes in weight quantization layers are initialized in the same way, but in activation quantization layers, the step sizes are initialized as Tensor(1.0). The authors use Tensor(v.abs().mean() * 2 / sqrt(Qp)) as initial values of the step sizes in both weight and activation quantization layers, where Qp is the upper bound of the quantization space, and v is the initial weight values or the first batch of activations. Initial Values of the Quantization Step Size My implementation generally follows the v2 version, except for the following points.
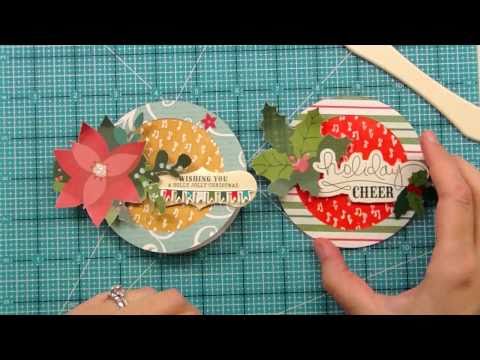
Recently they released a new version v3, which fixed some typos in the v2 version.
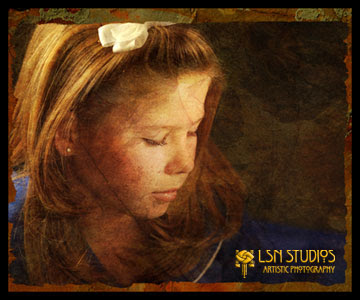
To improve accuracy, the authors expanded the quantization space in the v2 version. LSQ-Net paper has two versions, v1 and v2. Implementation Differences From the Original Paper You can find some example configuration files in the example folder. You can modify the option resume.path: /path/to/ in the YAML file to resume the training process, or evaluate the accuracy of the quantized model. After every epoch, the program will automatically store the best model parameters as a checkpoint. For details, please read the comments in config.yaml. The modified options in your YAML file will overwrite the default settings. Python main.py /path/to/your/config/file.yaml
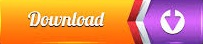